Research News
Jan 15, 2025
- Informatics
Reading signs: New method improves AI translation of sign language
Additional data can help differentiate subtle gestures, hand positions, facial expressions
Improving AI accuracy
Adding data such as hand and facial expressions, as well as skeletal information on the position of the hands relative to the body, to the information on the general movements of the signer’s upper body improves word recognition.
Credit: Osaka Metropolitan University
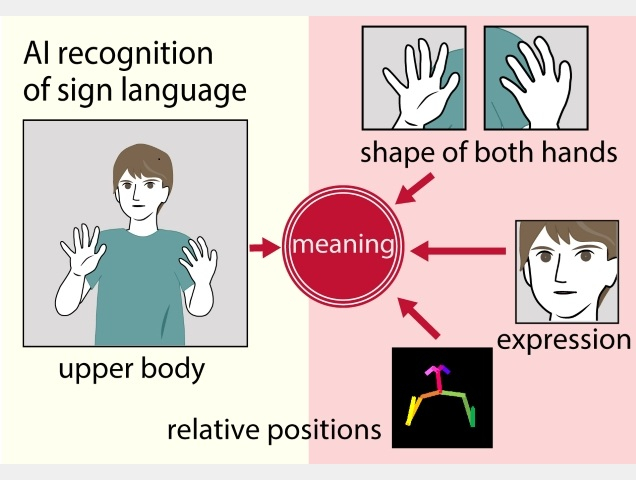
Sign languages have been developed by nations around the world to fit the local communication style, and each language consists of thousands of signs. This has made sign languages difficult to learn and understand. Using artificial intelligence to automatically translate the signs into words, known as word-level sign language recognition, has now gained a boost in accuracy through the work of an Osaka Metropolitan University-led research group.
Previous research methods have been focused on capturing information about the signer’s general movements. The problems in accuracy have stemmed from the different meanings that could arise based on the subtle differences in hand shape and relationship in the position of the hands and the body.
Graduate School of Informatics Associate Professor Katsufumi Inoue and Associate Professor Masakazu Iwamura worked with colleagues including at the Indian Institute of Technology Roorkee to improve AI recognition accuracy. They added data such as hand and facial expressions, as well as skeletal information on the position of the hands relative to the body, to the information on the general movements of the signer’s upper body.
“We were able to improve the accuracy of word-level sign language recognition by 10-15% compared to conventional methods,” Professor Inoue declared. “In addition, we expect that the method we have proposed can be applied to any sign language, hopefully leading to improved communication with speaking- and hearing-impaired people in various countries.”
The findings were published in IEEE Access.
Funding
This work was supported by JSPS KAKENHI JP19K12023.
Paper information
Journal: IEEE Access
Title: Word-Level Sign Language Recognition With Multi-Stream Neural Networks Focusing on Local Regions and Skeletal Information
DOI: 10.1109/ACCESS.2024.3494878
Authors: Mizuki Maruyama, Shrey Singh, Katsufumi Inoue, Partha Pratim Roy, Masakazu Iwamura, and Michifumi Yoshioka
Published: 11 November 2024
URL: https://doi.org/10.1109/ACCESS.2024.3494878
Contact
Graduate School of Informatics
Email: inoue[at]omu.ac.jp
*Please change [at] to @.
SDGs